The problem
Functional Magnetic Resonance Imaging (or fMRI) is a non-invasive neuroimaging technique that can be used to study brain function. The most common fMRI signal is the Blood-Oxygen-Level-Dependent signal (or BOLD signal), related to local changes in the concentration of deoxyhemoglobin in the blood.
In the past fifteen years, different variations of the widely used “Balloon model” have been introduced to provide a high-level description of the physiological processes underlying the hemodynamic response, from neuronal activation to the BOLD signal. The Balloon Model depends on several biophysical parameters (around 15), for which different competing values have been proposed in the literature. Most approaches currently use one of these empirical sets of values or perform the estimation using gradient-based approaches from the prior mean estimates (whose choice is not trivial). The BOLD data used were recorded to test biophysical models in the context of epileptic activity in rats.
Methods employed
In order to avoid the limitations of the procedure traditionally employed to estimate these biophysical parameters, we used an evolutionary computation method called Differential Evolution. Evolutionary Computation methods, part of the metaheuristics family, are population-based and general-purpose stochastic procedures designed to solve complex optimization problems where enumerative or gradient-based search methods are inappropriate. Evolutionary Computation methods require the definition of a fitness function to measure the goodness of the parameters found. We used Bayesian modeling to derive an appropriate fitness function. Also, to palliate the absence of ground truth in real data, a synthetic dataset was created to study the methods behavior under controlled conditions.
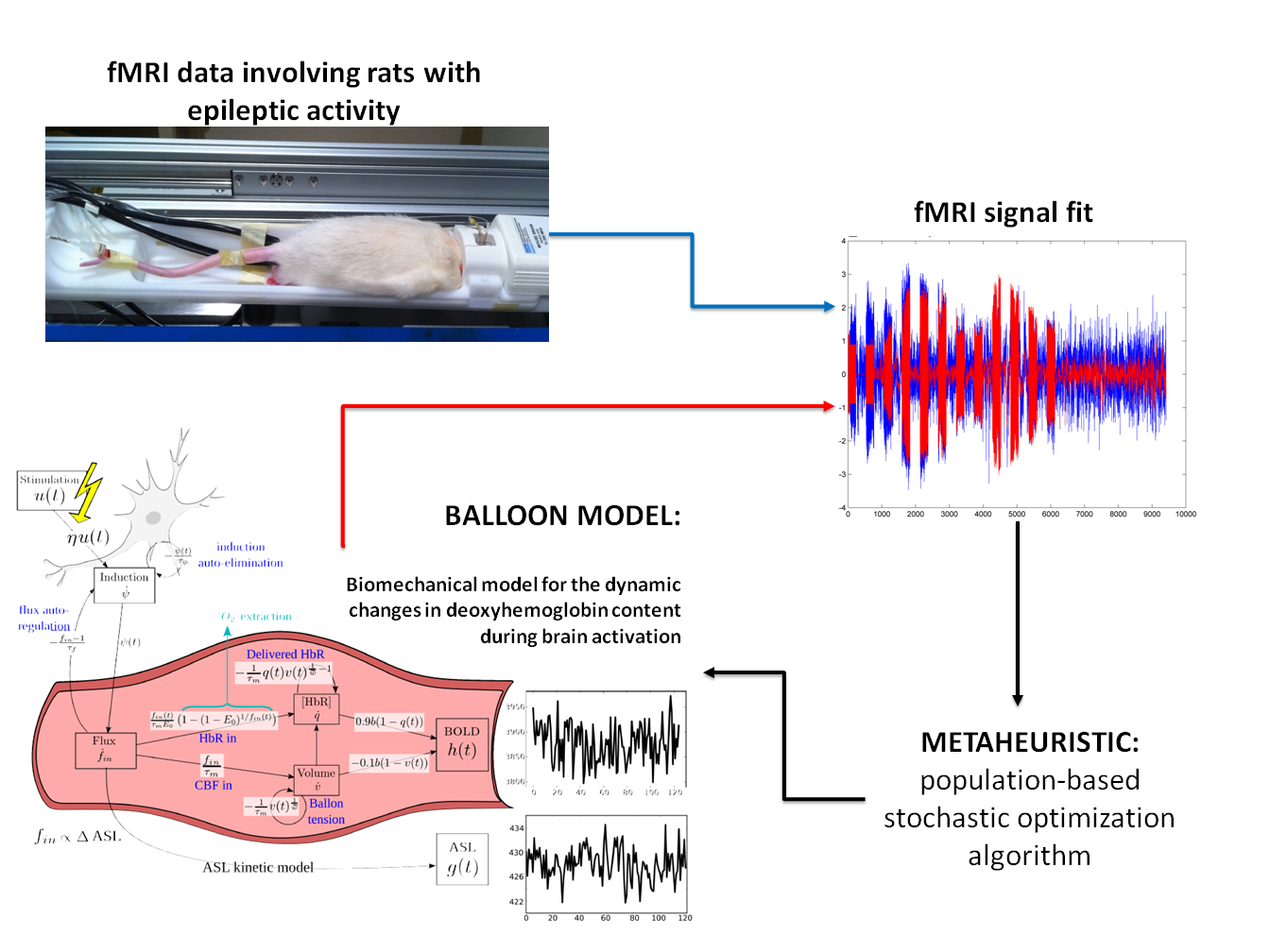
Results
A novel method to infer physiological parameters from observed BOLD signals was designed, implemented and validated (comparing it with previous approaches), showing the robustness and flexibility of global search optimization methods while being able to incorporate prior information in a principled Bayesian way. This method neither needs the provision of initial estimates nor the computation of gradients. The new approach provided better signal fitting in most real data sets. The estimates obtained for these parameters seemed both more realistic and more stable or at least as stable across sessions as the gradient-based estimates. Our results were published in reference journal in signal processing (Mesejo, P., Saillet, S., David, O., Bénar, C., Warnking, J., and Forbes, F. (2016), A Differential Evolution-based Approach for Fitting a Nonlinear Biophysical Model to fMRI BOLD Data, IEEE Journal of Selected Topics in Signal Processing 10 (2), 416-427) and at a top-rank medical imaging conference (MICCAI, possibly the most prestigious and competitive conference concerning medical image computing: Mesejo, P., Saillet, S., David, O., Bénar, C., Warnking, J., and Forbes, F., Estimating Biophysical Parameters from BOLD Signals through Evolutionary-based Optimization, 18th International Conference on Medical Image Computing and Computer Assisted Intervention (MICCAI’15), Part II, 528-535, Munich, 2015). The software developed was registered in France on 20/07/2016 (reference: IDDN.FR.001.300022.000.S.P.2016.000.31230. BOLD model FIT). This software was also released as a MATLAB toolbox at https://hal.inria.fr/hal-01221115v2/file/fMRImodelFIT_v1.zip.
Participants
Institutions
National Institute for Research in Computer Science and Automation (Institut National de Recherche en Informatique et en Automatique, Inria, France) and Grenoble Institute of Neurosciences (Grenoble Institut des Neurosciences, GIN, Francia). This work was funded through an Inria project entitled “Advanced statistical models for the coupling of ASL and BOLD MR modalities to study brain function” (62.904€).
Researchers
Dr. Pablo Mesejo as postdoctoral researcher at the MISTIS team (Modeling and inference of complex and structured stochastic systems), led by Dr. Florence Forbes, at Inria Grenoble Rhône-Alpes (Francia). This project was developed in close collaboration with Dr. Jan M. Warnking, researcher at the Grenoble Institute of Neurosciences (GIN).